What is Fit Finder?
Goal of Fit Finder technologies to help shoppers find the right size the first time to reduce the number of returned clothing and footwear products due to sizing to improve customer satisfaction and give a good reason to shop in stores that offer Fit Finder. And the bigger picture is sustainability, and the profitability of the business.
The Evolution of Fit Finder Technology
Due to the fascinating evolution of technology, we have moved way beyond those Basic Size Charts. Now retailers use Fit Finder technologies that use many data sources, different algorithms to try to predict the perfect size and fit for shoppers online.
The concept of a Fit Finder is not entirely new; however, its capabilities have been vastly enhanced over the years. Initially, these tools relied on basic algorithms that considered general measurements to suggest sizes. Today, advanced Fit Finders leverage sophisticated artificial intelligence and machine learning algorithms to provide highly accurate and personalised size recommendations. By analysing a shopper’s unique body measurements, preferences, and even past purchase behaviours, these tools can suggest sizes with remarkable precision.
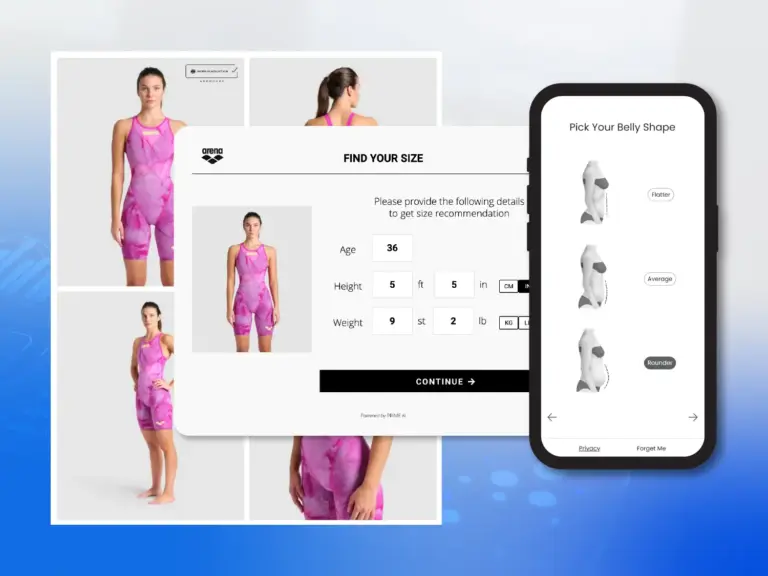
Fit Finder Types
The ultimate question is can Fit Finders actually solve the problem and can we say goodbye to those online refunds due to sizing – that’s the dream. We are breaking down a bunch of different types of Fit Finder technologies here, so you can think of them as a spectrum of complexity. So, on the simpler side we have Size Chart Comparative digital versions and on the most complex side we have Predictive AI with Adaptive Hybrid Systems.
Static Size Chart Comparators Digital Versions
Static size chart comparative digital versions of the size charts you find in every store. These are generic based on what the brand thinks should fit, but no relation to individual product style. Easy for retailers to implement some free apps on the Shopify App Store and other app stores, but these are not very personalised to the brand, nor to the shopper, and we are not even going into more variables that affect sizing. So, these are not very helpful and not groundbreaking, it is level one of fit finder technologies.
Basic Statistical Tools
Think of them as the predecessors to the more advanced systems. These types of tools on Shopify App store cost around $50 and less per month, despite various pricing models each has. This type of Fit Finder technology takes a few basic measurements into account, despite them asking about height, weight and some body shapes, it lacks the complexity and the predictive power of the fancier ones of the more advanced systems that use more sophisticated statistical methods or incorporate machine learning. So, they are not quite smart with limited data and no understanding about actual products or body shapes whatsoever. In more simple terms it’s like a calculator.
Brand to Brand Comparators
It’s like you looking at a size chart saying I am a medium in H&M, so I am a medium in this brand. It’s magic, right? In many cases you will find that your input is identical to the answer you get. This is not very sophisticated. As you know, each style and size can fit totally differently and even the same product in different colours can fit differently. It has been reported many times from shoppers and journalists, read here to see an example. In other words this type of Fit Finder is rubbish in, rubbish out. Some of them have elements of Machine Learning (which is labelled in marketing materials as AI) to bridge the gap between rubbish in, rubbish out, however it is impossible to bridge this gap as each product is unique on how it fits, how it stretches, in what style it should be worn etc. Then this needs thousands if not millions data points, by the time it collects this data even at global scale product reaches its end of season, meaning these technologies can never learn at fit level, and they are lightyears away from understanding product fit at Product ID level, and the colour level is even further away than lightyears, and then add in the mix of different sized people that are tall and thin, wide hips etc. You can see the limitations clearly already. Sizing is so inconsistent across different brands and even inside the brand making Brand to Brand Comparators, the results are very icy. Read here about H&Ms sizing inconsistency here.
Statistical Matching Tools
This is a step up in level of sophistication in Fit Finder technologies. These use data from a lot of shoppers to make size recommendations at category level, some try at product ID level, but product ID level is too challenging for this technology. They take thousands, millions of shoppers, associate things like weight, height, some body shape inputs, with the purchases and assume that other shoppers with the identical inputs might fit into the same size. Which is not the case in the first place, secondly these need millions of data points and these are typically available for well known brands and the most common sizes like S, M, L. Anything beyond these becomes a struggle and peppered with inaccuracies. This type of Fit Finder technology gets better over time as they analyse more data, but as mentioned, accuracy diminishes when going beyond people that are in the middle of the sizing spectrum. When going for fit, the product ID level results become very flaky, so there is no need to even discuss further about the capabilities at the colour level of such technology. These quite often claim to be Artificial Intelligence, this is where the grey zone starts about the definition of “What is AI?”.
Predictive AI with Hybrid Systems
We are talking about systems that leverage artificial intelligence and machine learning to create highly personalised size recommendations. Systems also gather information about age, weight, height, body shape form which can be outputted body measurements. AI analyses all information about the actual shoppers visiting specific online stores, past purchases. It learns purely from the actual customers in that store, not something that has happened in another country in another store with similar products. In other words, data is not clouded about history somewhere else. It purely learns from a single retailer’s actual data and if a retailer trades in multiple locales, the evolution is specific to the locale goods being traded. All this, along with brand specific size charts and it uses really complex algorithms to understand every single SKU from the actual inventory that the retailer holds, yet again not similar products. Since visually the same product produced in different factories or in a different year can fit differently and the same person would need a different size. Technology is like your personal shopping assistant that consciously learns at multi-level dimensions. Getting data from various sources, visual analysis, customer feedback, sales, refunds data, past purchases and more. Different sizing by category, fit and even product ID, despite everything looking the same at the front end, the customer in the back end, there are complex neural networks and adaptive AI systems orchestrating the process to determine the right size for the shopper. You can then imagine what insights you can get with the collected data to optimise the business. Do you know how much money you leave on the table? You not only unlock missed sales opportunities, but reduce operational costs due to less refunds, making your business more profitable. But these types of tools come with their own price tag. This is not your Shopify App store, a copy/paste app printed for every single retailer the same. This type of Fit Finder requires precision in connecting systems and the custom integration is carried out for every single client to adapt to their systems and data sources.
Body Scanning Solutions
Body scanning tools used to try and help these sizing issues have not been a popular choice amongst customers. The invasive process of having to upload images of your body to an app has left some customers feeling uneasy and uncomfortable. It is intrusive and too time consuming, thus pretty much no customers are using them. Read a case study about a retailer who moved from body scanning solution to Prime AI’s Fit Finder here. You can also read here to see the comparison of Prime AI’s Fit Finder vs. Body Scanning Solutions.
Why Use Fit Finder?
- Sustainability is increasingly important for all industries, online fashion is no exception. Think about all those returns, about those clothes being shipped back-and-forth. It creates a huge carbon footprint, so if technology can help even a small percentage of shoppers find the right fit the first time, the environmental impact becomes really substantial when this technology is adopted widely. It is good for the shoppers, it is good for the planet. Thus, having such technology for retailers should really be mandatory. Read more about benefits in McKinsey & Company report.
- Accurate sizing recommendations have led to a decrease in size-related returns, addressing issues like ‘bracketing’ where customers order multiple sizes to try on at home Vogue Business.
- Even Amazon decided to give more reasons for shoppers to shop on their marketplace by moving into the Fit Finder technology domain by switching off their try before you buy at home service – read here.