Prime AI Zero Input Sizing Solution
Prime AI Zero Input Sizing, what we call internally a V3 Size Finder is an AI-driven solution that addresses one of fashion e-commerce’s biggest pain points: finding the right size without any effort from the shopper. By leveraging zero-input machine learning and predictive AI, Prime AI automatically predicts the best fit for each customer without requiring them to take quizzes or enter measurements.
This frictionless approach improves the online shopping experience and boosts retailer KPIs. The solution’s value proposition is clear: it guides shoppers to the right size with high accuracy, helping retailers reduce costly returns and increase conversions. In trials and deployments of V3, Prime AI’s sizing recommendations had influence on more than 50% of all sales that led to over 30% fewer returns by customers who saw size advice vs. those who did not, and a 3 – 15% uplift in conversion rates, while providing a better purchasing experience. This translated to significant profit impact while improving customer loyalty.
Overall, Prime AI’s zero-input sizing offers a frictionless, data-driven solution that enhances customer confidence and profitability for clothing retailers.
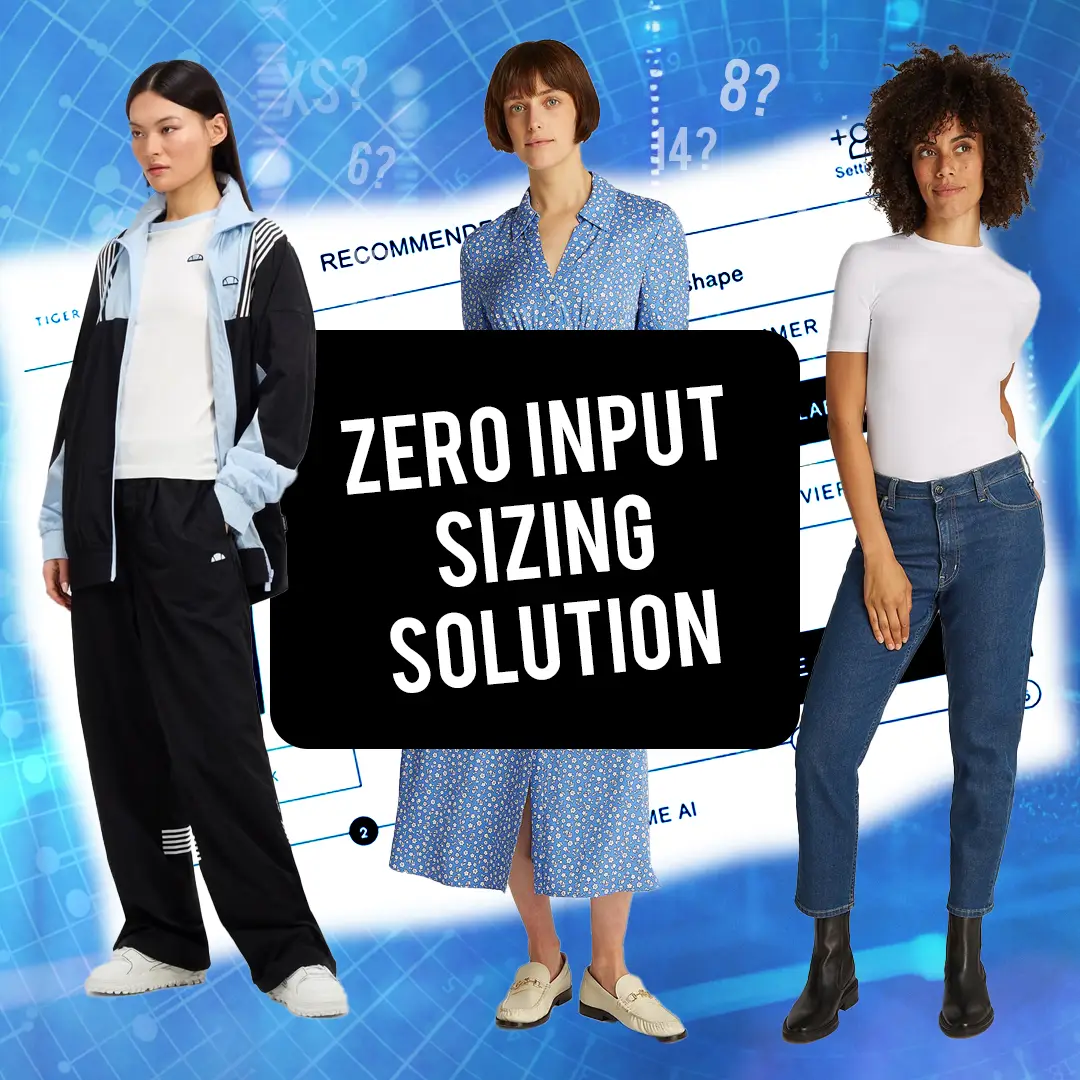
Online apparel retail has long struggled with sizing and fit challenges, leading to high return rates and dissatisfied customers. Industry analyses show that roughly 50% of shoppers’ online clothing purchases are returned due to size or fit issues. A common practice known as “bracketing” where consumers order the same item in multiple sizes and return what doesn’t fit has become widespread, driving up logistics costs and eroding margins.
These issues persist despite traditional tools like size charts and fit guides. Many e-commerce sites still provide only basic or confusing size charts, forcing customers to guess or measure themselves, which often results in mistakes. Early interactive sizing tools (such as fit quizzes) attempted to improve on static charts by asking shoppers about their body measurements or the sizes they wear in other brands.
While such quizzes showed that data driven guidance can reduce returns to a limited extent and often below retailers’ expectations. These quizzes have created some friction in the shopping journey requiring shoppers to input height, weight, or favorite clothing items before getting a recommendation.
Other methods like 3D body scanners or AR try-on technology have been piloted, but these often need extra hardware or user effort, limiting their adoption.
In short, traditional sizing solutions have limitations: they either rely on user effort (which deters some shoppers) or fail to personalise enough at the individual level. This has left a gap in the market for a more seamless approach. Retailers face not only direct costs from returns (handling a return can cost 3x the outbound shipping) but also intangible costs: environmental impact from reverse logistics and customer churn.
Studies indicate that poor fit and cumbersome return processes drive customers away. One survey found three-quarters of shoppers would avoid purchasing again from a retailer if they had a bad sizing experience. These challenges set the stage for Prime AI’s zero-input sizing solution, which emerged to overcome the drawbacks of earlier tools by combining accuracy with ultimate convenience.
Drawbacks of earlier solutions
Prime AI Technology Overview
Prime AI’s solution is powered by predictive Artificial Intelligence and machine learning techniques tailored to clothing sizing. At its core is a set of bespoke neural networks trained on each retailer’s and users data. Unlike simple rule-based size charts, these deep learning models ingest a rich array of inputs: purchase history, return behavior, product specifications, and fit feedback.
The Pro version of the system learns exclusively from the retailer’s actual inventory and product composition data, as well as from customer reviews, sales, and refunds records. By analyzing what sizes customers bought and whether those purchases were kept or returned (and why), the AI discerned patterns about which body shapes or dimensions each garment truly fits.
Importantly, Prime AI’s neural networks operate at the individual shopper and Product Sub Category level – delivering size predictions specific to each individual product style. This granularity means the system understands that a size “M” in one dress might not fit the same as a size “M” in another due to cut or fabric differences. Indeed, material properties like stretch, fabric weight, and cut are factored in by the models; the AI learns, for example, that a jersey cotton shirt might accommodate different body measurements than a non-stretch denim jacket.
Prime AI’s technology uses predictive modeling that goes beyond static tables, analysing real customer behaviour and product relationships to match each shopper with their best fit. It even employs natural language processing (specifically, Large Language Models) to mine customer reviews for insights automatically detecting comments about fit (e.g. “runs tight in the shoulders” or “fabric has no give”) to refine sizing predictions.
Additionally, Prime AI leverages collaborative filtering techniques (comparable to those used in content recommendation) to handle scenarios where data is sparse. By grouping shoppers with similar purchase and return profiles, and products with similar fitting patterns, the system can make intelligent size recommendations for first-time buyers or new products with limited direct history.
In summary, the Prime AI solution marries neural networks (for deep pattern recognition) with retailer-specific data (sales, returns, product specs) to create a self-learning sizing model that is far more dynamic and accurate than traditional methods.
How Prime AI Delivers Granular Accuracy
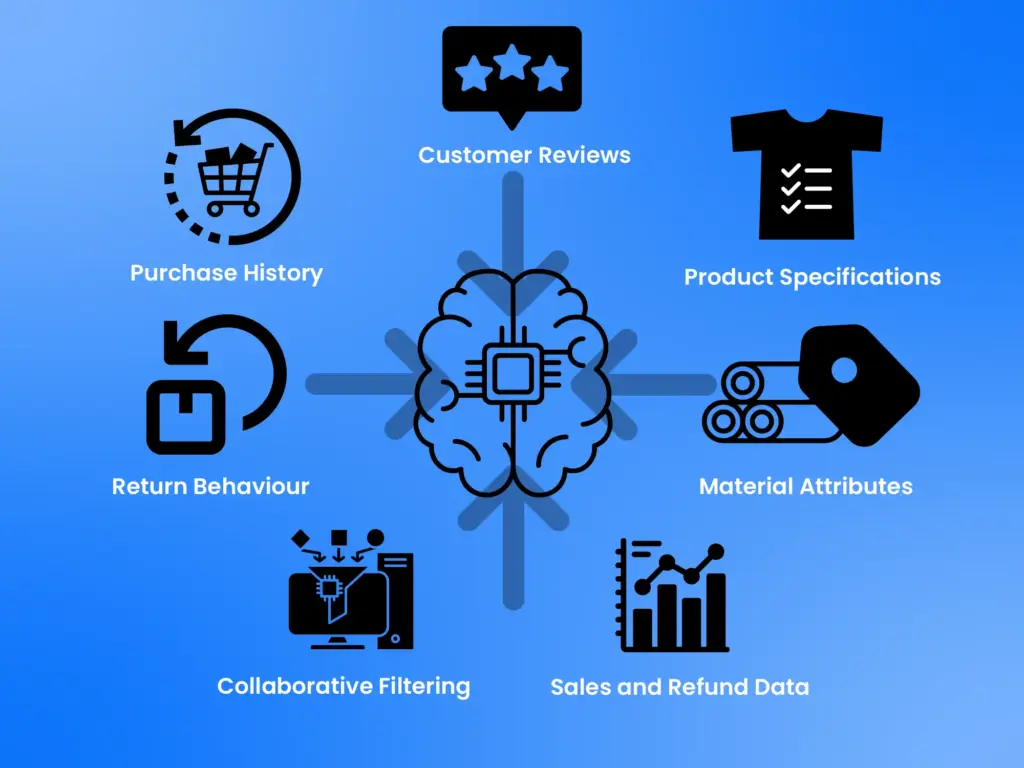
Frictionless Experience
A defining feature of Prime AI’s sizing solution is its zero-input, frictionless design. This means shoppers get size recommendations instantly and without filling out any forms or questionnaires. The moment a customer views a product, the system can suggest the best size for them (e.g. “Recommended size: Medium”) based on the data in the background.
Eliminating the usual fit quiz or measurement form creates a smoother user journey, one where the customer isn’t interrupted by extra steps. This design is particularly valuable given modern consumers’ short attention spans and preference for quick, seamless shopping on mobile devices.
Research shows that when faced with uncertainty about size, customers either delay purchase, seek help, or resort to bracketing. By removing the uncertainty instantly, Prime AI’s tool keeps shoppers moving toward checkout.
The interface is typically integrated right into the product page, showing a suggestion (and sometimes a confidence indicator or explanation) in real-time. For example, a returning shopper might see “Recommended size: 36” displayed next to the size options, without any action needed on their part.
Prime AI’s interface provides an instant size recommendation (“Recommended size: 36”) without requiring the user to input personal measurements. Removing quizzes and forms creates a frictionless experience that guides the shopper to the right fit intuitively. As illustrated above, the customer simply selects the suggested size and proceeds, trusting the AI’s guidance. This streamlined process has significant benefits: it reduces drop-off rates (since customers are not tempted to abandon their cart out of sizing confusion or form fatigue) and instills confidence that they are ordering the correct size. In fact, even though Prime AI offers an optional quiz mode for those who prefer it, most retailers find the adoption of the zero-input mode to be very high when enabled. By harnessing data behind the scenes, the solution creates a personalised fitting-room effect online with zero effort from the shopper, truly living up to the promise of a frictionless customer experience.
Frictionless Zero Input Recommendations
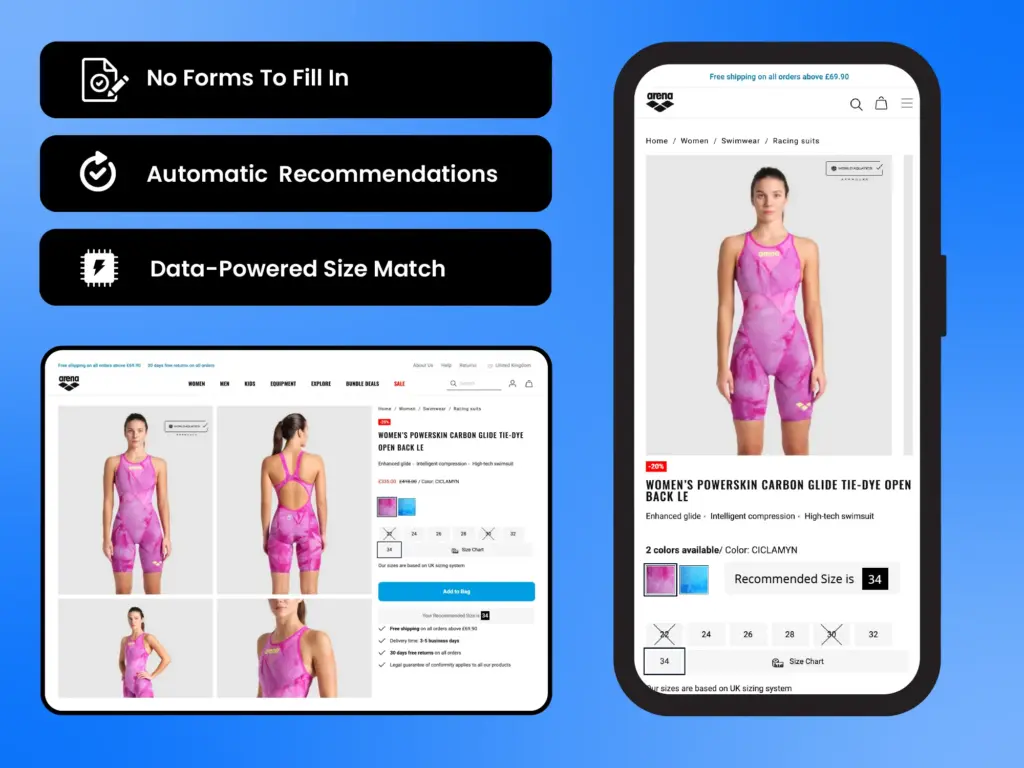
Business Impact
The Prime AI zero-input sizing solution drives measurable improvements in key business metrics for clothing and footwear retailers. By guiding customers to the right fit the first time, it effectively boosts conversion rates and reduces returns, directly impacting the bottom line.
Retail case studies show that implementing Prime AI’s size recommendations can reduce return rates by a significant margin on the order of 31% fewer returns for shoppers who use the recommended size versus those who do not. Fewer returns translate to saved costs in reverse logistics, less inventory churn, and more satisfied customers.
Moreover, retailers see a notable conversion uplift. By removing hesitation around sizing, the solution increases the likelihood that browsers become buyers. Conversion rate improvements ranging from +3% up to +15% after deploying the tool. Even a single-digit percentage increase in conversion can mean millions in additional revenue for large retailers, so this impact is substantial.
Importantly, these benefits are not confined to a small subset of shoppers; the majority of customers are touched by the feature. In fact, over 50% of all sales on a site can involve an AI-driven size advice when Prime AI’s PRO integration is fully utilized. This indicates high customer engagement with the recommendations (largely because they are automatic and unobtrusive) and shows that the solution scales across the user base.
Engagement is measured by monitoring how many shoppers order the recommended size and how this behaviour is impacted by various dynamic messages related to sizing. Beyond the hard numbers of conversion and returns, there are qualitative business impacts as well. Retailers often observe higher average order values and increased shopper loyalty. When customers trust that a retailer will get their size right, they are more likely to complete purchases and come back for repeat orders.
The first-time buyer still has some friction, to answer the quiz to allow Prime AI to start building their profile. This reduces the chance of a poor first fit which might dissuade them from returning. Industry data underscores that a negative fit experience can turn a large portion of customers away from a brand. By improving first-purchase success rates, Prime AI helps convert one-time visitors into loyal customers.
Additionally, by curbing “size sampling” behaviors (where customers intentionally over-order multiple sizes), the solution also protects margins and inventory. In summary, Prime AI’s zero-input sizing delivers a compelling ROI through higher conversion, lower return costs, and better customer lifetime value.
As one fashion retailer found, shoppers who received accurate fit recommendations not only bought more frequently, but their conversion rate more than doubled compared to those not using the tool, a testament to the transformative impact of AI-guided sizing on e-commerce performance.
See how Clothing Size Finder Pro has helped brands slash returns
Continuous Improvement
A key strength of Prime AI’s solution is that it continuously learns and improves over time. The system operates on a feedback loop: every new purchase, return, exchange, or review provides additional data to refine the sizing models. After deployment, the AI quickly adjusts to the specific customer base and product catalog of the retailer. It “learns fast from sales, returns, customer reviews, and other data” automatically.
For example, if the model initially predicts a size L for a particular dress but a significant number of customers return that dress citing it was “too large,” the algorithm will incorporate that outcome. It may learn that the dress runs big and start recommending size M to similar customers in the future. This dynamic recalibration happens behind the scenes, ensuring that recommendations get smarter with each transaction.
The incorporation of customer review analysis is another powerful feedback mechanism. As Prime AI’s system ingests more free-form text from reviews (using LLM technology), it can detect recurring fit issues or praises (e.g. “tight in arms, order one size up”) and automatically adjust recommendations or at least flag those nuances in the dynamic messaging.
In effect, the solution crowdsources fit feedback at scale and feeds it into the predictive model.
Furthermore, Prime AI’s collaborative filtering approach allows it to make predictions even in new scenarios by drawing on analogies from existing data. If a completely new product is introduced with no sales history, the system can compare its attributes to similar items in the catalog and leverage the “wisdom of the crowd” from those to guess the right sizes for the new item.
Similarly, for a brand-new shopper with no profile or purchase history, Prime AI can match that user to clusters of similar shoppers (based on broad behavior or referral source, etc.) to provide an initial size estimate instead of showing nothing. As more data specific to that user comes in, the recommendations become more precise. The machine learning models are retrained and fine-tuned regularly to incorporate the latest data patterns.
Prime AI provides retailers with analytics dashboards that show fit recommendation performance and areas of improvement, which can also inform manual adjustments or business decisions (for example, if many customers sized out of a certain range are trying to buy, merchandising could adjust the size range in the future).
Over time, this virtuous cycle of data -> prediction -> outcome -> data means the sizing advice stays current with evolving trends (such as new fashion cuts, seasonal changes in purchasing, or shifting customer demographics).
In summary, the longer Prime AI’s solution runs, the smarter and more accurate it becomes, continuously reducing error rates and improving customer satisfaction. This adaptive learning capability ensures that the sizing recommendations don’t grow stale and that retailers see sustained and even increasing benefits the more the system is used.
Challenges and Limitations
User trust and education, some shoppers might ignore or mistrust the recommendation initially, especially if it suggests a size that differs from their self-image or usual choice. They might default to old habits (like buying two sizes anyway). Overcoming this requires clear communication (e.g. showing a dynamic message like “Smaller sizes than recommended are returned more often” to build trust). Also, the solution’s effectiveness is tied to customers shopping while logged in or identifiable (to access their history). If a large portion of users shop anonymously or as guests, it limits the data available for zero-input recommendations. Retailers may need to incentivise account creation or data linking to maximize the system’s coverage.
Future Outlook
The area of growth is leveraging wearable technology. As wearable devices (smartwatches, smart belts, etc.) gather fitness and body data (like body dimensions, weight fluctuations, posture), in the future such information (with user consent) could feed into sizing algorithms. For example, a connected fitness app could update a user’s current measurements seamlessly into their profile.
In terms of algorithmic advancement, future sizing solutions will likely use even more advanced neural network architectures and training techniques. We might incorporate reinforcement learning, where the AI experiments with recommendations and gets reward signals based on return outcomes to continuously self-optimize.
On the user experience front, expect the process to become even more user-friendly and proactive. Instead of a size recommendation only on the product page, future systems might integrate with personal shopping assistants or bots. A customer could receive size guidance in a chatbot conversation or even voice assistants (e.g., “Alexa, what size should I order this jacket in?” and Alexa responds based on your Prime AI profile). There’s also room for integrating social and stylistic feedback – e.g., combining AI sizing with style preference AI to say “Size L would fit you best for a standard fit. For an oversized streetwear look, consider XL.” From a retailer’s perspective, these AI tools will increasingly contribute to product development and inventory planning. The aggregated data on how different sizes fit various customers can inform design adjustments,some brands using Prime AI’s data to tweak future designs and inventory quantity by size.
Finally, as shoppers’ expectations evolve, they may come to expect near-perfect fit guidance as a standard part of online shopping. This will drive more retailers to adopt these tools, and competition will spur improvements. The outlook includes more personalisation, cross-channel integration (using the same size profile in physical store), and the blending of AI sizing with sustainability efforts by reducing returns, these tools contribute to environmental goals, which will be a strong narrative in future retail strategies.
In essence, AI sizing solutions will become more accurate, more holistic, and more interconnected with other technologies.
Prime AI’s zero-input system today is likely just the beginning of the future iterations that could make the dream of “online shopping with perfect fit, every time” a reality through a combination of data intelligence and emerging tech innovations.